Latest Benefits of Accounts Payable Audit in 2024: Unlocking Unexpected Funds for This Year’s Budget
In the ever-evolving landscape of business finance, maintaining a robust and agile budget is crucial. One often overlooked but highly beneficial strategy to achieve this is through an accounts payable (AP) audit. In 2024, the benefits of conducting an AP audit have become more pronounced, particularly in terms of short-term recovery of unexpected funds. Here’s a closer look at how an AP audit can be a game-changer for your organization’s budget this year.
1. Identification of Overpayments and Duplicate Payments
One of the primary advantages of an AP audit is the identification and recovery of overpayments and duplicate payments. In the hustle of managing numerous transactions, errors can easily slip through the cracks. An AP audit meticulously reviews past payments, uncovering instances where vendors were paid more than once or amounts that exceed the actual invoice. Recovering these funds can provide a significant boost to your budget in the short term, freeing up capital that can be reallocated to pressing needs.
2. Recovery of Unclaimed Credits and Discounts
Businesses often miss out on credits and discounts due to oversight or administrative errors. An AP audit helps in identifying these unclaimed credits, early payment discounts, or rebates that your organization is entitled to but hasn’t utilized. By reclaiming these, you can inject immediate funds back into your budget, enhancing liquidity and financial flexibility.
3. Detection of Fraudulent Activities
Fraud in accounts payable can drain a company’s resources unnoticed. An AP audit can detect irregularities and fraudulent activities, such as fictitious vendors or inflated invoices. Identifying and addressing fraud not only helps in recovering lost funds but also strengthens internal controls, preventing future occurrences. This proactive approach safeguards your budget against unforeseen financial drain.
4. Enhancement of Vendor Relationships
Through the process of an AP audit, discrepancies and payment errors that could strain vendor relationships are identified and corrected. Clear communication and resolution of these issues foster trust and collaboration with vendors. In some cases, this may lead to renegotiation of terms, resulting in better pricing or payment terms that benefit your budget in the short term.
5. Streamlining of Processes and Cost Savings
An AP audit often reveals inefficiencies in the payment process. By addressing these inefficiencies, organizations can streamline their AP processes, resulting in cost savings. These savings, while initially small, can accumulate over time, providing additional funds for your budget. Improved processes also mean fewer errors in the future, reducing the likelihood of unplanned financial outflows.
6. Compliance and Risk Management
Ensuring compliance with regulatory requirements is essential for avoiding fines and penalties. An AP audit helps in verifying adherence to tax regulations and other compliance mandates. By identifying and correcting non-compliance issues, organizations can avoid costly penalties, thus preserving budgetary allocations for strategic initiatives.
7. Improved Cash Flow Management
An AP audit provides a clear picture of your financial obligations and cash flow. By identifying due payments, pending invoices, and any discrepancies, organizations can better manage their cash flow. Improved cash flow management means that funds are available when needed, preventing cash crunches and allowing for smoother budget execution throughout the year.
Latest Benefits of Accounts Payable Audit in 2024: Uncover Hidden Funds for Your Year-End Budget
In the fast-paced business landscape of 2024, every penny counts. With economic uncertainties and tightening budgets, companies are seeking innovative ways to optimize their finances. One often overlooked strategy is the accounts payable (AP) audit – a powerful tool that can unearth hidden funds and provide a much-needed boost to your year-end budget.
Why Accounts Payable Audits Matter Now More Than Ever
AP audits delve deep into your company's payment processes, meticulously examining invoices, contracts, and financial records. The primary goal is to identify errors, discrepancies, and potential overpayments that may have slipped through the cracks. In the current economic climate, recovering these funds can make a significant difference in your bottom line.
Short-Term Recovery: The Key to Immediate Financial Relief
While AP audits offer a range of long-term benefits, their ability to provide short-term financial relief is particularly relevant in 2024. Here's how:
- Uncover Overpayments: Audits frequently reveal duplicate payments, incorrect calculations, or payments made for goods or services not received. Recovering these overpayments can inject a significant amount of cash back into your budget.
- Identify Fraudulent Activity: AP audits can expose fraudulent invoices or unauthorized payments, safeguarding your company's assets and preventing further financial losses.
- Reclaim Unclaimed Credits: Your company may be entitled to credits from suppliers for various reasons, such as early payment discounts or pricing errors. An audit can help you track down these credits and apply them to your current financial obligations.
- Negotiate Better Terms: Armed with detailed data from the audit, you can negotiate more favorable terms with suppliers, potentially reducing your costs and freeing up additional funds.
2024 Budget Impact: Realizing the Benefits
The funds recovered through an AP audit can be strategically allocated to bolster your year-end budget. Consider using these unexpected resources for:
- Cash Flow Improvement: Address immediate financial needs, such as payroll, operating expenses, or debt repayment.
- Strategic Investments: Fund projects that were previously on hold due to budget constraints.
- Emergency Reserves: Build a financial cushion to protect your company from unforeseen challenges.
Take Action Today
Don't let hidden funds slip away. An accounts payable audit is a proven method for recovering unexpected resources and strengthening your financial position in 2024. Whether you conduct an in-house audit or partner with a specialized firm, the potential benefits far outweigh the investment.
Conclusion
In 2024, the benefits of an accounts payable audit extend far beyond traditional financial oversight. The ability to recover unexpected funds and optimize budgetary allocations makes AP audits an indispensable tool for organizations looking to enhance their financial health. By uncovering hidden opportunities and mitigating risks, an AP audit ensures that your budget remains robust and agile, ready to meet the demands of the year ahead. Implementing regular AP audits can thus be a strategic move, unlocking immediate financial gains and setting the stage for sustained fiscal stability.
Act now to unlock the hidden value in your accounts payable and secure your company's financial future.
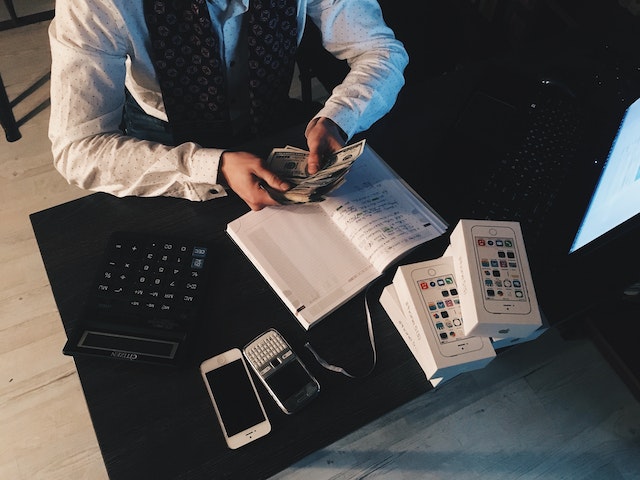
How Could AI Automation Encourage Duplicate Payments to Vendors?
Top of Page
AI automation, while beneficial in many ways, can sometimes lead to issues such as duplicate payments to vendors. Here's how AI automation could potentially create these problems:
1. Data Entry Errors
Automated systems rely heavily on accurate data inputs. If the initial data entry contains errors, such as duplicate invoice numbers or incorrect vendor details, AI systems might process these as separate entities, leading to duplicate payments.
2. Lack of Integration Between Systems
AI systems often operate across multiple platforms and databases. If there is a lack of proper integration and synchronization between these systems, the same invoice might be submitted and processed multiple times, resulting in duplicate payments.
3. Incorrect Algorithm Configuration
AI systems are guided by algorithms that dictate how they process data. If these algorithms are incorrectly configured, they might not recognize duplicate invoices. For instance, if the matching criteria for detecting duplicates are too lenient, slight variations in invoice numbers or vendor names might bypass the system's checks.
4. Overlapping Automation Rules
Multiple automation rules or workflows can sometimes overlap, causing the same invoice to be processed more than once. For example, if one rule is set to pay invoices within a certain period and another is triggered by a specific vendor’s invoice submission, both might end up processing the same invoice separately.
5. Human Override of Automated Processes
In some cases, human intervention can inadvertently cause duplicate payments. If an employee manually processes an invoice that has already been handled by the AI system, or overrides a flag raised by the system indicating a potential duplicate, this can lead to duplicate payments.
6. Errors in Vendor Communication
Automated systems sometimes rely on communications with vendors for confirmations and updates. If a vendor submits an invoice multiple times due to miscommunication or system errors, and the AI system doesn't properly track these submissions, it could process each submission as a new invoice.
7. Mismanagement of Invoice Status
AI systems track the status of invoices from submission to payment. If there are errors in updating the status of invoices, an invoice marked as unpaid might be processed again, resulting in duplicate payments. This can happen due to system lags, crashes, or bugs in the software.
8. Inadequate Duplicate Detection Mechanisms
While AI can be designed to detect duplicates, the effectiveness of these mechanisms depends on how well they are implemented. Inadequate or poorly designed duplicate detection algorithms might miss duplicates, especially if the duplicate invoices have minor differences or if the data is inconsistently formatted.
While AI automation can streamline and improve efficiency in accounts payable processes, it can also introduce risks that lead to duplicate payments if not implemented and managed carefully. Here are some scenarios where AI automation could contribute to duplicate payments:
- Data Input Errors:
- Incorrect Data Entry: If the AI model is trained on inaccurate or incomplete data, it might misinterpret invoice details, leading to duplicate entries and payments.
- Data Duplication: Errors in data integration or migration between systems could cause invoices to be duplicated within the AI system, triggering duplicate payments.
- Algorithm Flaws:
- Overly Sensitive Matching: If the AI algorithm is too sensitive in matching invoices, it might flag slight variations (e.g., typos, formatting differences) as separate invoices, leading to duplicate payments.
- Under-Trained Models: If the AI model hasn't been trained on a sufficiently diverse dataset, it might struggle to accurately identify and distinguish duplicate invoices from legitimate ones.
- Integration Issues:
- Lack of Synchronization: If the AI system isn't properly synchronized with other financial systems (e.g., ERP), there might be a delay in updating payment records, potentially causing the AI to process the same invoice multiple times.
- System Glitches: Technical errors or malfunctions in the AI system or its integration with other platforms could lead to unintended duplication of payment requests.
- Human Oversight:
- Overreliance on Automation: While AI can automate tasks, it's crucial to maintain human oversight. If human reviewers fail to catch errors made by the AI system, duplicate payments could slip through.
- Lack of Training: If staff are not properly trained on how to use and monitor the AI system, they might inadvertently trigger duplicate payments or fail to identify errors.
Mitigating the Risks:
To prevent AI automation from causing duplicate payments, companies should:
- Invest in High-Quality Data: Ensure that the AI system is trained on accurate, clean, and comprehensive data.
- Refine Algorithms: Continuously refine and improve the AI algorithms to enhance their accuracy in identifying duplicates.
- Establish Robust Integration: Ensure seamless integration between the AI system and other financial platforms to maintain data consistency.
- Maintain Human Oversight: Implement a system of checks and balances where human reviewers verify the AI's decisions before payments are processed.
- Provide Adequate Training: Train staff on how to use the AI system effectively and how to identify and address potential errors.
Conclusion
AI automation has the potential to streamline and improve many aspects of accounts payable processes. However, without proper oversight, integration, and algorithm configuration, it can inadvertently lead to issues such as duplicate payments. Regular audits, continuous improvement of AI algorithms, and ensuring robust integration between systems are essential to mitigate these risks and harness the full potential of AI in accounts payable automation. By taking these proactive measures, businesses can harness the benefits of AI automation while minimizing the risk of duplicate payments and protecting their financial resources.
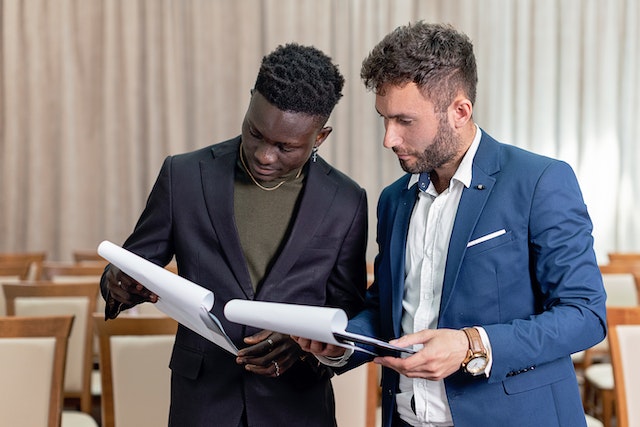
Could AIs Begin Generating Errors Undetectable to Humans?
Top of Page
As AI systems become more sophisticated, their potential for generating errors that are difficult for humans to detect increases. Here's how:
-
Complexity of AI Models: Deep learning models, like those used in natural language processing or image generation, are inherently complex. They make decisions based on intricate patterns and correlations that may not be easily understandable to humans. This complexity can lead to errors that are subtle and difficult to trace.
-
Adversarial Attacks: AI systems can be vulnerable to adversarial attacks, where malicious actors intentionally manipulate input data to cause the AI to make mistakes. These attacks can be designed to exploit the specific weaknesses of an AI model, making the resulting errors very difficult for humans to detect.
-
Data Biases: AI models learn from the data they are trained on. If this data contains biases, the AI will inherit those biases and generate outputs that reflect them. These biases can lead to errors that are discriminatory or unfair, but may not be immediately obvious to humans.
-
Over-Reliance on Automation: As AI systems become more integrated into various processes, there's a risk of over-reliance on their output. If humans become too trusting of AI and stop actively checking its work, subtle errors could go unnoticed.
-
Evolution of AI Capabilities: AI is constantly evolving, and new capabilities are being developed at a rapid pace. As AI systems become better at mimicking human behavior, their ability to generate convincing but incorrect outputs will also improve.
Examples of Undetectable Errors:
- Subtle Textual Errors: AI-generated text could contain factual errors, misrepresentations, or logical inconsistencies that are not immediately obvious to a casual reader.
- Hidden Biases in Decision-Making: AI algorithms used for decision-making could incorporate hidden biases that lead to unfair or discriminatory outcomes, even if the AI's reasoning seems sound on the surface.
- Deepfakes and Misinformation: AI-generated images, videos, or audio could be used to create convincing deepfakes or spread misinformation that is difficult to distinguish from real content.
Mitigating the Risks:
To address the risks of undetectable AI errors, we need to:
- Prioritize Explainability: Develop AI models that are more transparent and explainable, so their decision-making processes can be better understood and audited.
- Robust Testing and Validation: Rigorously test and validate AI systems to identify and address potential vulnerabilities before they are deployed.
- Human Oversight and Collaboration: Maintain strong human oversight of AI systems, ensuring that humans are actively involved in the decision-making process and are able to identify and correct errors.
- Ethical Considerations: Incorporate ethical considerations into the development and deployment of AI systems, ensuring that they are designed to be fair, transparent, and accountable.
AI systems, especially those employing machine learning and other advanced techniques, can indeed generate errors that humans might find difficult to detect. These errors can arise from several factors inherent in the complexity and opacity of AI operations. Here’s how AI could produce undetectable errors and what this implies for human oversight:
1. Algorithmic Complexity and Black Box Nature
Many AI algorithms, particularly deep learning models, operate as "black boxes." Their decision-making processes are not easily interpretable by humans. This opacity can obscure errors, making it challenging for humans to understand where and why mistakes occur. For instance, subtle biases in training data might lead to biased outputs, which are hard to trace without deep technical analysis.
2. Subtle Data Corruption
AI systems process vast amounts of data and can sometimes propagate subtle errors within this data. These errors might not be immediately obvious and could accumulate over time, affecting the AI’s performance in ways that are not easily noticeable. For example, minor inaccuracies in financial data processing might go undetected but could lead to significant discrepancies over time.
3. Misinterpretation of Context
AI systems can misinterpret context or nuances in data that humans might easily understand. For example, natural language processing (NLP) models might misunderstand idiomatic expressions or cultural references, leading to erroneous outputs. These mistakes can be subtle and not easily detected by human reviewers unless they closely analyze the context.
4. Sophisticated Adversarial Attacks
Adversarial attacks involve intentionally designed inputs that exploit the weaknesses of AI models, causing them to make errors. These inputs are often imperceptibly different from normal inputs to human observers but can significantly impact AI behavior. Detecting and defending against such sophisticated attacks requires advanced technical expertise.
5. Overfitting and Underfitting
AI models can overfit or underfit training data, leading to errors when processing new, unseen data. Overfitting means the model is too closely tailored to the training data and fails to generalize, while underfitting means the model hasn’t learned enough from the data. These issues might not be immediately apparent, especially if the AI’s performance appears adequate on surface-level metrics.
6. Bias and Fairness Issues
AI systems trained on biased data can perpetuate or even amplify these biases, resulting in unfair or discriminatory outcomes. Such biases can be deeply embedded in the model’s functioning and difficult to detect without specific fairness audits and bias detection mechanisms.
7. Complex Interdependencies in Data
In complex systems, there can be intricate interdependencies between different data points. AI might make errors when these interdependencies are not fully understood or represented in the model. These errors can be hard to detect because they depend on the interaction between multiple variables, which may not be obvious during routine checks.
Mitigation Strategies
To mitigate the risk of undetectable errors in AI systems, several strategies can be employed:
- Transparency and Explainability: Developing AI models with greater transparency and explainability helps humans understand how decisions are made, making it easier to detect and correct errors.
- Regular Audits: Conducting regular, thorough audits of AI systems, including bias detection and fairness audits, can help identify subtle errors.
- Robust Testing: Implementing extensive testing regimes that include edge cases and adversarial scenarios to uncover potential weaknesses.
- Human-in-the-Loop: Incorporating human oversight in AI decision-making processes ensures that AI outputs are reviewed and validated, particularly in critical applications.
- Continuous Monitoring: Employing continuous monitoring and feedback loops to detect anomalies and errors in real-time.
Conclusion
While AI offers significant advantages in efficiency and capability, it also introduces the risk of generating complex errors that humans might find hard to detect. Addressing this challenge requires a combination of technical solutions and rigorous oversight to ensure the reliability and fairness of AI systems. By taking these steps, we can harness the power of AI while mitigating the risks of undetectable errors and ensuring that AI technology serves humanity's best interests.
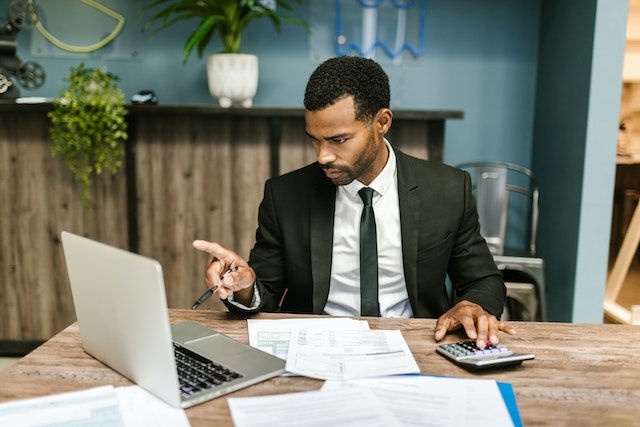
AIs Have Learned from Journals Anaylzed by Humans
Top of Page
Indeed, AI systems have learned and continue to learn from a vast array of sources, including journals analyzed and written by humans. This process involves leveraging human-generated knowledge to train models and improve their capabilities. Here’s how this learning from human-analyzed journals can influence AI and the implications it carries:
1. Incorporation of Expertise
AI systems benefit from the depth of knowledge contained in academic journals. These journals are typically peer-reviewed, meaning they contain vetted, high-quality information. By training on such data, AI can acquire a nuanced understanding of complex subjects, improving its analytical capabilities and decision-making processes.
2. Advancement in Specific Fields
AI systems can specialize in specific fields by learning from specialized journals. For instance, AI trained on medical journals can assist in diagnosing diseases or suggesting treatments based on the latest research findings. This specialization enhances the utility and accuracy of AI in specific domains.
3. Emulation of Human Analytical Skills
Journals often contain not just data, but also the analytical processes used by researchers to draw conclusions. AI can learn these processes, emulating the critical thinking and analytical skills of human experts. This capability allows AI to perform complex analyses, generate hypotheses, and provide insights that mimic human expertise.
4. Biases and Limitations
However, AI learning from human-analyzed journals also inherits the biases and limitations of these sources. Academic journals can reflect the prevailing biases of the time, including cultural, gender, and methodological biases. If not addressed, these biases can be perpetuated and even amplified by AI systems.
5. Updating and Relearning
AI systems must continually update and relearn to stay current with the latest research. Academic knowledge is constantly evolving, and new findings can overturn previous understandings. Continuous learning mechanisms are necessary to ensure that AI remains relevant and accurate in its analyses.
6. Error Detection and Correction
The meticulous nature of academic research provides a strong foundation for training AI, but it’s not foolproof. AI systems must be equipped with mechanisms to detect and correct errors in the data they learn from. This includes identifying retracted papers, correcting for flawed methodologies, and adjusting for contradictory findings across different studies.
7. Ethical and Responsible AI
Learning from journals also highlights the need for ethical and responsible AI development. This involves ensuring that AI systems respect the integrity of the research process, properly attribute sources, and maintain transparency in how they use and interpret academic knowledge.
Conclusion
Leveraging Human Wisdom
AI's learning from human-analyzed journals signifies a significant advancement in harnessing human wisdom. These journals, rich with peer-reviewed, high-quality information, enable AI systems to acquire a deep, nuanced understanding of complex subjects, enhancing their analytical capabilities and decision-making processes.
Enhancing Specialization
Through specialized journals, AI can develop expertise in specific fields, offering valuable insights and assistance. For instance, AI trained on medical journals can aid in diagnosing diseases and suggesting treatments based on the latest research findings, demonstrating its potential to become a specialized tool in various domains.
Emulating Human Analysis
By analyzing the critical thinking and analytical processes found in journals, AI can emulate human expertise. This ability allows AI to perform complex analyses, generate hypotheses, and provide insights that closely mimic human analytical skills, further bridging the gap between machine learning and human intelligence.
Addressing Biases and Limitations
While AI benefits from the wealth of knowledge in journals, it also inherits the biases and limitations present in these sources. Academic journals can reflect prevailing cultural, gender, and methodological biases. Without proper mitigation, these biases can be perpetuated and amplified by AI systems, emphasizing the need for bias detection and correction mechanisms.
Continuous Learning
To remain relevant and accurate, AI systems must continuously update and relearn from the latest research. The dynamic nature of academic knowledge requires AI to adapt to new findings, ensuring its analyses and recommendations are based on the most current information.
Ethical and Responsible AI Development
Learning from journals underscores the importance of ethical and responsible AI development. AI systems must respect the research process's integrity, attribute sources correctly, and maintain transparency in using and interpreting academic knowledge. This approach ensures AI systems are reliable, trustworthy, and aligned with ethical standards. While it's true that AI models are often trained on large datasets that include human-analyzed journals, this doesn't entirely eliminate the risk of AI generating errors that humans may not detect. Here's why:
-
Limited Data and Bias: Even the largest datasets are still a limited sample of all possible information. Human biases present in the analyzed journals can be inadvertently incorporated into AI models, leading to errors that perpetuate those biases. AI might miss subtle nuances or misinterpret information, leading to conclusions that deviate from human understanding.
-
Evolving Knowledge: Human understanding and knowledge constantly evolve. Information that was considered accurate in the past might be outdated or proven incorrect later. If an AI model is trained on older data, it may not reflect the latest findings and could generate errors based on outdated knowledge.
-
Complexity Beyond Human Comprehension: AI models, especially deep learning models, often operate in ways that are difficult for humans to fully grasp. They can identify complex patterns and correlations in data that may not be immediately apparent to human analysts. This complexity can lead to unexpected errors that are hard for humans to predict or diagnose.
-
Overfitting and Generalization: AI models can sometimes overfit the training data, meaning they perform well on the specific data they were trained on but fail to generalize to new, unseen data. This can lead to errors when the AI encounters situations or information that differ from its training data.
-
Lack of Contextual Understanding: While AI can process vast amounts of information, it may lack the deeper contextual understanding that humans possess. This can lead to errors in interpreting information, especially in situations that require nuanced understanding of cultural, social, or historical contexts.
Conclusion
In conclusion, AI systems learning from human-analyzed journals represent a significant step forward in leveraging human knowledge. This learning process enables AI to enhance its analytical capabilities, specialize in various fields, and emulate human expertise. However, it also necessitates addressing biases, ensuring continuous learning, and upholding ethical standards. By doing so, we can harness the full potential of AI while mitigating its risks, creating a powerful tool for advancing knowledge and solving complex problems. Even though AIs learn from human-analyzed journals, it's crucial to remember that AI is still a tool that requires human oversight and validation. It's essential to continuously monitor AI-generated outputs, identify potential errors, and provide feedback to refine the models.